Attribution Modeling vs. Marketing Mix Modeling: Which is Right for Your Online Business?
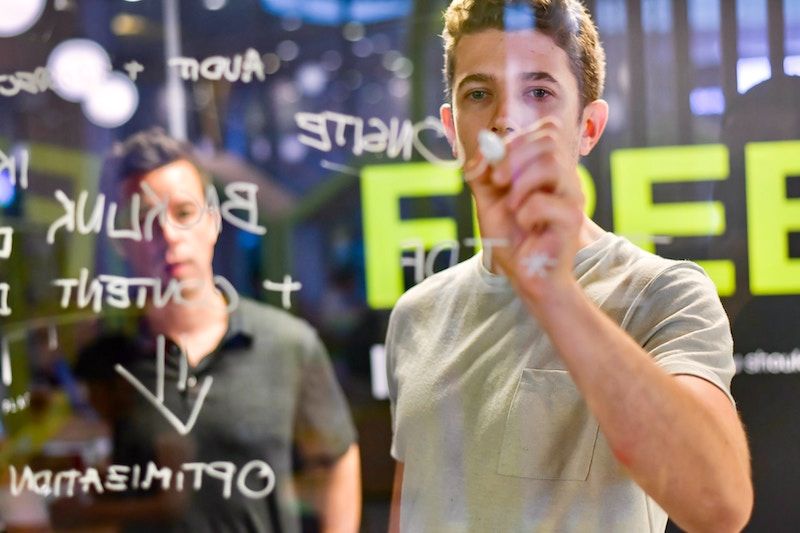
In the fast-changing environment, most companies are preoccupied with determining which marketing approach is the most fruitful. Although there are countless marketing platforms at your hand and clients can be found just about everywhere, organizations must evaluate which channels will provide the strongest results and return on investment.
Marketing mix modeling (MMM) has been around for quite some time. Organizations that sell fast-moving consumer goods often apply this approach, and it was one of the few instruments for integrated marketing research with financial recommendations before the internet era.
In contrast, attribution modeling emerged alongside the world of digital marketing. It's the go-to method for online businesses where the consumer journey happens mostly or entirely online.
This article will explore the difference between marketing mix modeling and attribution modeling to help you decide which one is better for your business.
Defining attribution modeling
Attribution modeling is a bottom-up technique of evaluating marketing performance. It examines each phase of the conversion cycle to determine the worth of every project element.
This strategy arose from the demands and challenges of online sales, where massive amounts of information are gathered and processed. As a result, attribution modeling is typically used to track online shopping, promotion, and other conversion initiatives. As more advertisers combine their online and offline networks, attribution models evolve and develop a comprehensive picture to compensate for offline engagements that are more difficult to track.
Because attribution modeling utilizes a detailed approach, input is analyzed regularly and instantly.
Attribution modeling: PROS
Marketing budget optimization: Attribution models inform which channels generate the most engagements, allowing companies to allocate their marketing resources better. This helps marketing professionals to make necessary adjustments to the budget.
Improved product creation: Advertisers can effectively identify their customers' demands using person-level attribution. These findings can then be used to make product improvements that prioritize the characteristics that buyers want.
Higher ROI: Good attribution helps marketers target the relevant customer at the right moment with the perfect offer, resulting in better sales and higher ROI.
Better personalization: Businesses can use attribution intelligence to better determine the content and channels specific consumers prefer, letting them focus on the things they like during the whole user journey.
Attribution modeling: CONS
Attribution measures only clicks: Because attribution models often track just clicks, they fail to take into account every encounter along the consumer journey. To put it another way, most models ignore significant offline interactions, dark social, or reactions. Was your employee a guest during a high-profile webinar? Did you run into a Reddit post where someone praised your product? Not measurable.
False positivity: Many attribution models presume that each interaction positively influences the purchasing decision. This is troubling since people may purchase goods or services with which they have had negative experiences, leading to false positivity.
The deceptive assurance of complete traceability: You can't stay on top of every single person's impressions and interactions. and there's a good chance you never will because of legal, social, or logistical concerns. In reality, with the cookie's demise and the release of iOS 14, the list of measurable touchpoints has decreased, making attribution much more problematic. The issue here is that you may overvalue the sources you can actually measure. That's why you can find yourself making some poor judgments that overlook the most crucial parts of your client experience.
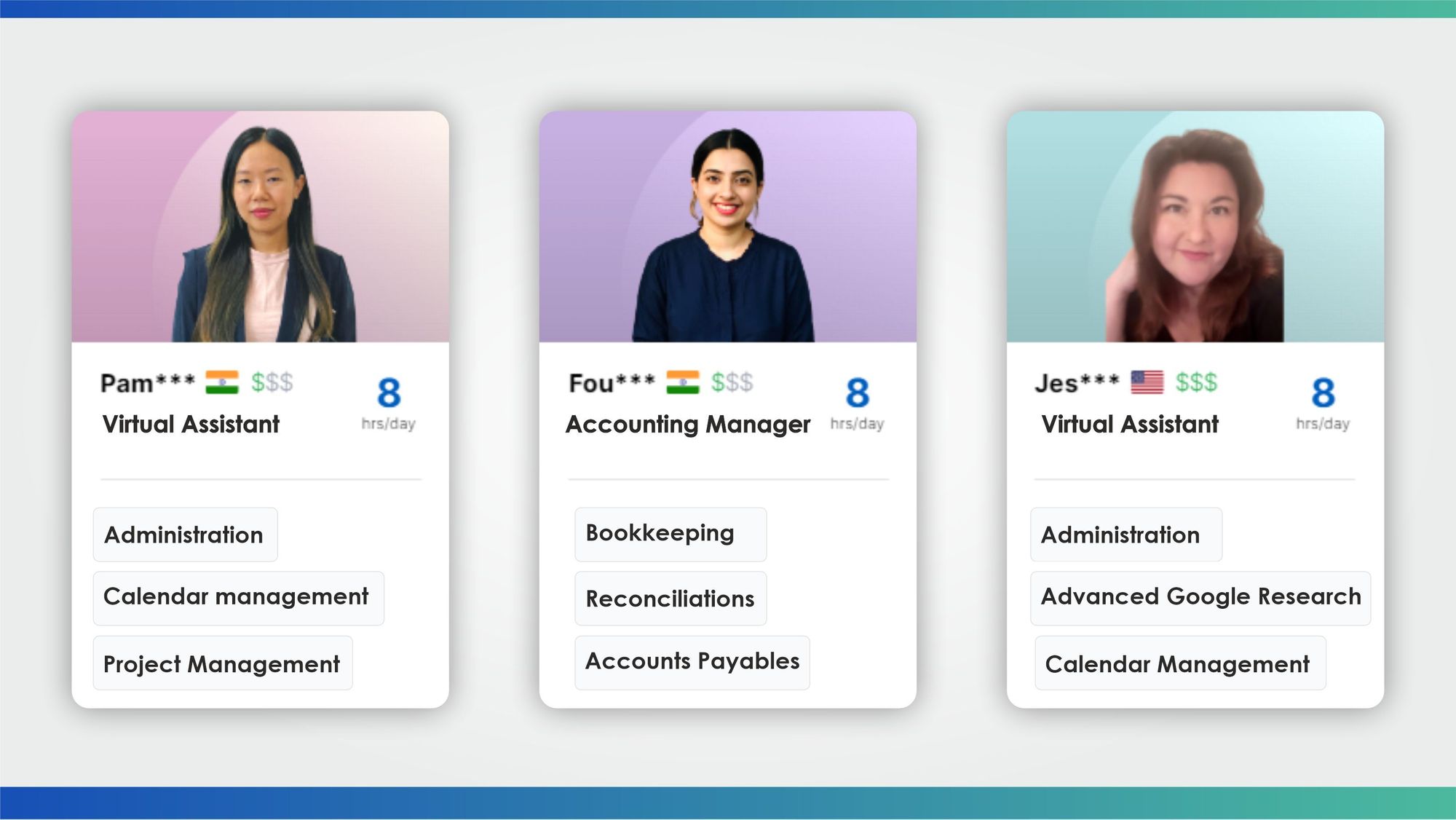
Defining marketing mix modeling
Rather than emphasizing user interactions, marketing mix modeling adopts a top-down approach to everything. It takes into account marketing and sales data, benchmarks, earnings, expenses, and external elements like demand and market situations, profitability, competition, and everything else that can influence customer behavior.
The marketing mix model evaluates four essential marketing components: pricing, product, promotion, and place. It aims to find the best mix of these four factors to meet a company's goals. It accomplishes so by collecting data from all of the variables that potentially influence the performance of marketing platforms and running a multiple regression.
Marketing mix modeling: PROS
Use knowledge to increase marketing efficiency: Traditional ways of monitoring campaign success are costly and tedious. Marketing experts may use marketing mix modeling to acquire data-driven suggestions and deliver lucrative outcomes. This sophisticated analytics methodology assists firms in fast obtaining insights while also speeding up tactical decision-making.
Analyzes each channel: Marketing mix modeling employs statistical approaches to study the inputs (like funding and outlets) in proportion to the outputs (like income or brand recognition), rather than relying on a shaky path of measurable consumer activities. Therefore, the intention is not to create a detailed model of the user journey but to grasp each channel's and activity's function.
Provides a full picture: Organizations can use MMMs to get a complete picture of their marketing performance and estimate marketing ROI. The technique examines both external and internal elements that influence traditional (and now also digital) channels. This method evaluates the marketing mix elements more precisely because it combines the ongoing and future attribution of the variables.
Marketing mix modeling: CONS
It demonstrates correlation rather than causality: Your model may show that sales went up by 25% while your TV campaign was in motion, but you can't establish that the sales growth was due to the TV ads.
You'll need a sizable budget to make MMM work: The biggest problem with MMM is that it only makes sense if your organization has grown to a particular size and has a considerable media budget. It's because, in order to truly measure the effect of every platform and campaign, you must establish diversity in your marketing mix.Slow-shifting: MMM has been put to great use in the past for refining marketing initiatives, but it simply cannot provide the person-level, detailed information that today's marketers require. Marketing is constantly moving forward, and since that happens so quickly, long-term data gathering is not as reliable as it once was. This was noticeable during the COVID-19 outbreak, which required advertisers to instantly adapt to customer behavior alterations.
What is the main difference?
Different goals and priorities
Attribution modeling can be seen as a subcategory of MMM that focuses on comprehending and determining the best mix of 'online' sales channels - emphasis on the word 'online.'
Marketing mix modeling implementation is far more complicated than attribution modeling implementation.
Implementing attribution modeling is very simple because it rarely requires an 'end user' to do the statistics directly. The attribution model does the counting by itself. As a result, it does not require knowledge in statistics.
Attribution modeling gives you a lot more power over how you optimize your online communication for ROI.
The issue with marketing mix modeling is that it came before the internet. The idea was created when there was no online advertising and no browsers. It just couldn't keep up with the internet era.
Mix it up...
The two angles can have one drawback: if we take one way, we could ignore some of the elements contributing to our revenue. While MMM lets us reallocate resources to the most profitable channels, digital channels data are mostly left behind. We don't obtain some essential details about how the user experience unfolded during online interactions, nor do we identify how online factors influenced shopping choices. We're still missing data from the attribution modeling to address these queries.
Furthermore, we can't pinpoint any external variables that resulted in the consumer's initial interaction with our brand based on attribution. Digital attribution often does not cover baseline purchases. Every (favorable) interaction with our company appears to be a triumph of our web marketing efforts. However, many external factors - such as buying power, timing, and trends, among others - contribute to their success.
...but not just for the sake of it.
Many organizations combine both techniques and deal with two different data sources – one batch of macro facts from MMM and one collection of microdata from digital attribution modeling – to maximize the benefits and balance the drawbacks. However, instead of hybridization, this could result in misinterpretations, which could cause more harm than benefit. A chaotic mixture of two modelings is not viable, especially considering it requires twice the effort.
The best option is a combination of both models in a unified collection of information - a marketing mix attribution modeling. This method generates comprehensive observations and an overview of the various channels, as well as their connections and effects on customer behavior. Offline activities are no more tracked independently but rather in conjunction with the client data gathered through the attribution method.
Wrapping it up
Marketing mix and attribution modeling are tied to a much larger and more significant concept in marketing known as customer behavior modeling. Though they may appear simple, each has some sophisticated features that every strategist, innovator, and company owner should grasp before implementing them in their own business.
Despite their limitations, both models can be quite beneficial to a company's overall marketing strategy, and both methods can help you implement effective macro and micro improvements. Providing greater details on user behavior can help advertisers make effective financial planning judgments and maximize revenue.